Yi-Shiuan Tung
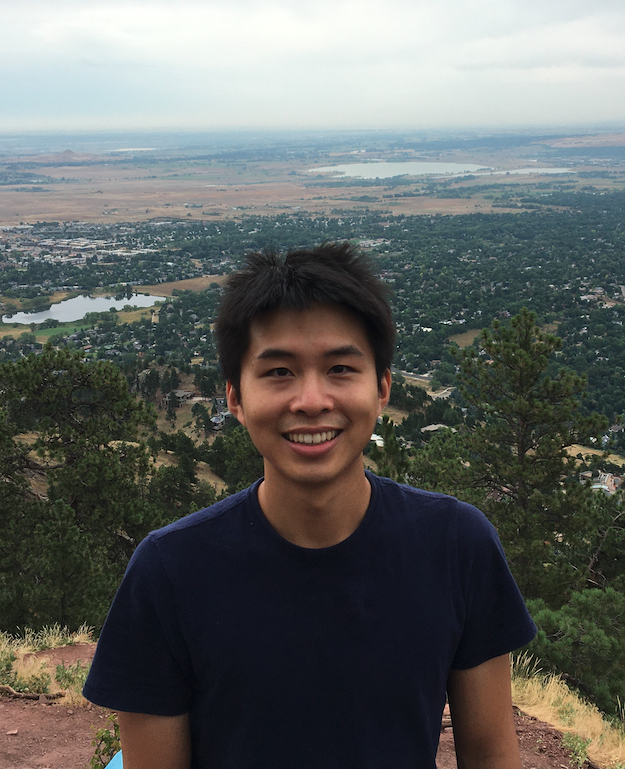
1111 Engineering Dr. ECES 111
Computer Science Dept, University of Colorado
Boulder, 80309 CO, USA
I am a PhD Student at CU Boulder co-advised by Professor Alessandro Roncone and Professor Bradley Hayes. My research interests are in leveraging environment design to enable fluid coordination among human-robot teams.
Previously, I worked with Professor Julie Shah at MIT on flexible assembly lines for human-robot collaboration. I received my Masters in Engineering and Bachelors Degree in Computer Science at MIT in 2018 and 2015 respectively.
news
Mar 13, 2024 | Presented our paper on Workspace Optimization Techniques to Improve Prediction of Human Motion During Human-Robot Collaboration at the HRI conference in Boulder! |
---|---|
Mar 13, 2023 | Presented our work on Improving Human Legibility in Collaborative Robot Tasks through Augmented Reality and Workspace Preparation at the VAM-HRI workshop! |
Aug 29, 2022 | Presented our paper on Bilevel Optimization for Just-in-Time Robotic Kitting and Delivery via Adaptive Task Segmentation and Scheduling at RO-MAN in Naples! |